The third edition of the International Workshop on Reading Music Systems (WoRMS) was held in a hybrid live/virtual setting on Friday, 23rd of July 2021. It brought together researchers and industry professionals working in Optical Music Recognition (OMR). This edition featured 11 papers on a variety of topics and an outstanding keynote from Anthony Wilkes (Organum Ltd) discussing the design of ReadScoreLib.
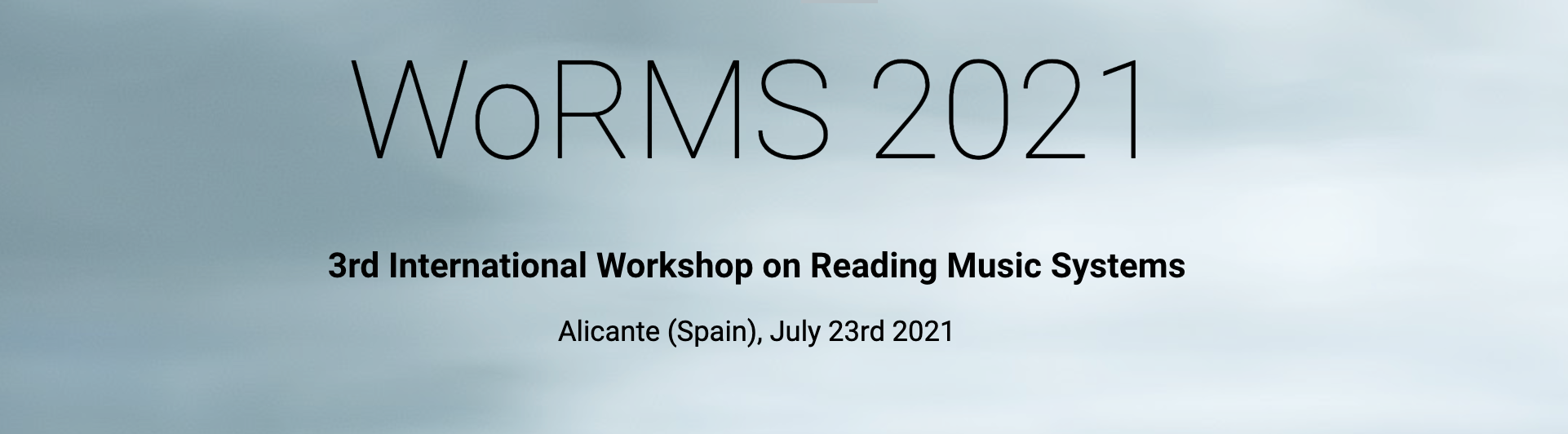
Below are summaries of some of the presented papers:
Hybrid Annotation Systems for Music Transcription
This paper explores combining human annotation and automated methods for music transcription. Results showed that audio extracts enhanced performance, particularly for short music segments.
Neural Network for Handwritten Melodies
Aimed at digital archiving for the University Library of Regensburg, this paper evaluates neural networks for recognising handwritten monophonic scores. Challenges include limited annotated data.
DoReMi: A Universal OMR Dataset
Our work presents DoReMi, a new dataset aimed at addressing challenges in OMR. We demonstrated baseline experiments using Faster R-CNN models for object detection.
Reconstructing Digits in Music Scores
This research highlights challenges in recognising and reconstructing digits in sheet music, achieving limited accuracy on real-world scores despite using deep learning.
Detecting Staves and Measures with Deep Learning
This paper investigates strategies to detect structural elements in music scores. Faster R-CNNs performed best for detecting system measures and staves.
Unsupervised Neural Document Analysis
This study proposes combining Domain Adaptation with Selectional Auto-Encoders for unsupervised document analysis, slightly improving state-of-the-art results.
Multimodal Audio and Image Music Transcription
This proof-of-concept explores combining OMR and AMT predictions, demonstrating slight improvements in error rates for monophonic scores.
Sequential Next-Symbol Prediction
This paper introduces a sequential classification-based approach to music scores using CNNs, addressing training set limitations.
Agnostic Transcription and Machine Encoding
This work focuses on encoding outputs from OMR into machine-readable formats using Machine Translation techniques.